PhD Studentship
Developing a novel experimental design and analysis model for longitudinal animal studies with high-dimensional outcomes
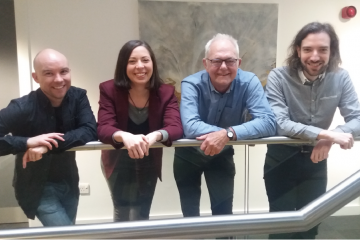
At a glance
Completed
Award date
September 2017 - December 2021
Grant amount
£90,000
Principal investigator
Professor Peter Diggle
Co-investigator(s)
Institute
Lancaster University
R
- Reduction
Read the abstract
View the grant profile on GtR