Data, data, data: How improving in vivo data management is good for the 3Rs
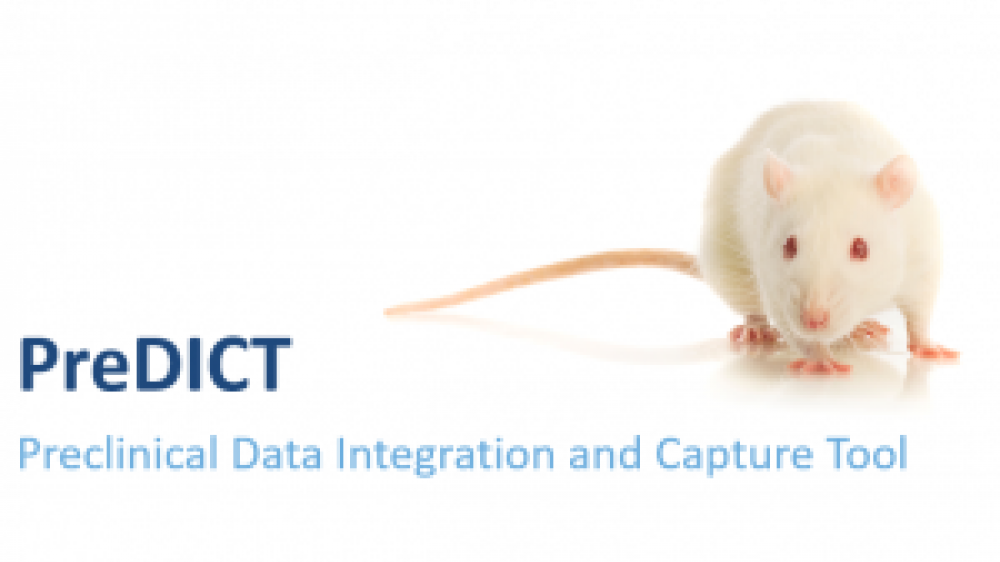
The second blog post in our series ‘Data, data, data’* is by Owen Jones from AstraZeneca. Owen describes their work on the PreDICT project to improve data management from in vivo experiments and reduce the use of animals in its research.
Rethinking preclinical data collection
In the world of pharmaceutical R&D, data from clinical trials is managed extremely rigorously – patient safety has to be monitored carefully, strict regulatory standards apply and data protection and privacy laws must be followed. It might be surprising, then, that relatively few standards and tools exist to support the capture and management of preclinical in vivo data, despite it often being just as complex and varied as clinical trial data.
This is starting to change as more and more pharmaceutical companies are embedding data science, systems biology approaches and predictive modelling into their R&D pipelines in their efforts to improve confidence in candidate drugs and avoid costly late-stage clinical failures. This has sharpened the industry’s appreciation that experimental data is a strategic asset that needs to be actively managed. Preclinical in vivo data is particularly critical as it plays a major role in making decisions about whether and how a drug will be administered to humans in clinical trials.
More than a spreadsheet
Clearly, in vivo scientists want to ensure that the complexity of their experiments is captured fully. However, since in vivo experiments are painstakingly tailored to the specific scientific questions they are designed to answer, many scientists feel that the only tools flexible enough to represent all the required nuances are spreadsheets, and are sceptical that more structured tools can do the job as well.
But there are obvious downsides to taking a case-by-case spreadsheet approach. Firstly, in vivo work often requires collaboration across multiple specialist disciplines, so data from a single study is very often broken up across several files. Working with data in this form can be very time-consuming and scientists need to take extra care to avoid introducing manual errors. Secondly, a great deal of attention and discipline is required to manage file-based data to ensure it can be found again when needed later. Finally, should the original custom file need to be referenced, the original author often needs to provide input to ensure all the details are appreciated and understood. There is therefore quite an overhead in actively managing file stores so that the need to repeat experiments is avoided.
In the AstraZeneca PreDICT programme, we first developed a set of data standards capable of clearly and fully representing in vivo study data from any research area. Having done that, we built PreDICT (Preclinical Data Integration and Capture Tool) itself – a central, global in vivo data store and data management platform that embeds these standards into every step of the data lifecycle.
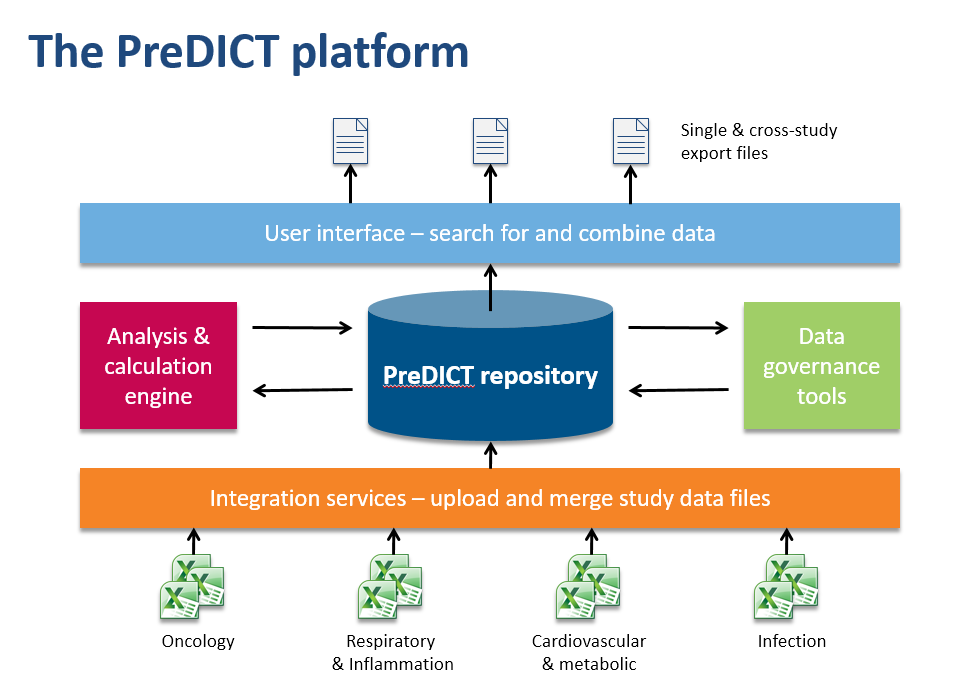
Making data processing more efficient
It might at first sound as if complying with new data standards would require even more time from scientists, but because PreDICT’s tools automatically take care of much of the attentive stewardship of data that previously had to be performed manually, we could deploy them in such a way that overall they considerably reduced the amount of time spent on administration. For example, PreDICT automatically merges data files from the separate disciplines into a single record of the study. This frees up scientists to concentrate on innovation and science rather than low-value data processing.
Scientists can now browse and search the entire body of in vivo study data in the centralised database, regardless of which disease area or R&D site generated it. Once found, data sets can easily be combined and exported in a convenient format to the user’s favourite tools for generating charts and reports or working on predictive models.
This really delivers a step change to AstraZeneca in terms of efficiency, data quality and what we could think of as the organisation’s institutional memory. Before PreDICT, searching through network drives full of spreadsheet files took time, and even when the right file was found the initial study team would often have to spend time advising on its correct use and interpretation. Now, the full experimental context of a data set is always captured alongside the results, and finding and formatting high-quality and readily understandable data with PreDICT is almost instantaneous.
Data scientists and modellers have reported overall time savings of around 30% from the easy availability of data in PreDICT. In one example, a Phase III project team needed to review all the relevant preclinical data for a compound that had been tested as a combination partner across multiple drug projects. To manually collate all that data from the original spreadsheet files would have taken at least a couple of weeks, but with PreDICT we completed the task in less than five minutes.
PreDICTing the 3Rs benefits
But PreDICT has not only provided efficiency and cost savings for AstraZeneca. Improving the management, quality and availability of in vivo data has a positive impact on each of the principles of the 3Rs.
Replacement – having quick access to high quality, unambiguous data makes it far easier for data scientists to develop predictive in silico models of how drugs behave in real organisms, and for them to have more confidence in the results of their work. In some cases, these models’ predictions might be good enough to replace in vivo experimentation altogether, but even where this isn’t the case, a good model might well be able to indicate when a proposed experiment has a high chance of failure (and therefore lead to the experiment being cancelled or redesigned). Overall, the better in vivo data is looked after, the more quickly the industry can move towards a future where reliably predictive computer models take the place of in vivo experimentation.
Reduction – the ability to draw on a large body of historical data helps scientists to optimise experimental designs to ensure that they are robust and that the maximum amount of knowledge is obtained per animal used. Having an easily searchable archive of historical data also helps to avoid accidentally repeating in vivo work that has been done before, and may also assist in reducing the number of animals used in new studies, for example by reusing control group data from comparable past studies.
Refinement – using the database means we can assemble data sets that allow large scale meta-analysis of animal welfare endpoints from many studies at once. And while organisations are required to carefully track and report the number and type of animals they have used in studies, a system like PreDICT allows them to gain deeper insight into how they were used – in which types of study design, for example – potentially opening further opportunities to improve overall welfare.
By investing in technologies to support faster and more efficient data analysis such as PreDICT, we can accelerate our goal of translating our groundbreaking science into life-changing medicines. As part of our transparent, collaborative approach to sharing our expertise and unique research tools, a customisable version of PreDICT is now being made available to other organisations so that they can share the benefits at a much lower cost. Contact us and our integration partners at info@predictsystem.com if you’d like to know more or organise a demonstration session or webinar for you and your colleagues.
*Other blogs in the series: