In silico human-based methodologies for evaluation of drug cardiac safety and efficacy
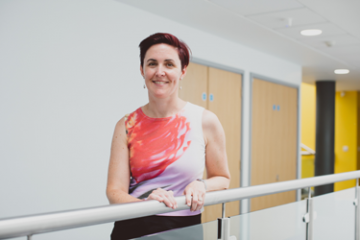
At a glance
Co-investigator(s)
R
- Replacement
- Reduction
Contents
Application abstract
Cardiotoxicity is one of the leading causes of failure during drug development and also, more worrying, after marketing approval. Withdrawal due to cardiotoxicity has increased from 5.1 to 33%, including compounds to treat cardiovascular problems as well as drugs not intended to affect the heart such as antihistamines. Current used strategy to screen for adverse contractility effects involves a combination of preclinical in vitro pharmacological profiling, cardiomyocyte assays and in vivo cardiovascular (CVS) studies, and uses a variety of animal species (rats, mice, rabbits, guinea-pigs, dogs, pigs and non-human primates).
In spite of the variety of animal methods for preclinical screening for drug safety, 20-50% of all advanced candidates have to be abandoned due to adverse outcomes, even late in the drug development process.
The main aim is to accelerate the uptake of human-based in silico methodologies for evaluation of cardiac drug safety and efficacy in industry, regulatory and clinical settings.
The specific objectives include:
1) Review, collation and implementation of a comprehensive database of human electrophysiology and contractility in silico multiscale mechanistic models for specific cardiac disease conditions.
2) Development and qualification of in silico human models for the prediction of adverse outcomes in human cardiac electrophysiology and contractility for specific disease conditions, based on existing models, and calibration with in vivo and ex situ recordings.
3) Evaluation studies to compare in silico human-based predictions to clinical outcomes, current animal methods, and in vitro methods including stem cell derived cardiomyocytes.
4) Workshops and dissemination activities to identify and overcome barriers for the uptake of in silico methods in industrial, clinical and regulatory settings.
Project membership involves key partners across 11 countries who will raise the profile of in silico human models for the 3Rs.
Impacts
Publications
- Riebel LL et al. (2024). In silico evaluation of cell therapy in acute versus chronic infarction: role of automaticity, heterogeneity and Purkinje in human. Scientific Reports 14:21584. doi: 10.1038/s41598-024-67951-5
- Zhou X et al. (2024). Lower diastolic tension may be indicative of higher proarrhythmic propensity in failing human cardiomyocytes. Scientific Reports 14:17351. doi: 10.1038/s41598-024-65249-0
- Musuamba FT et al. (2021). Scientific and regulatory evaluation of mechanistic in silico drug and disease models in drug development: Building model credibility. CPT: Pharmacometrics & Systems Pharmacology 10(8): 804–25. doi: 10.1002/psp4.12669
- Delaunois A et al. (2021). Applying the CiPA approach to evaluate cardiac proarrhythmia risk of some antimalarials used off-label in the first wave of COVID-19. Clinical and Translational Science 14(3): 1133-1146. doi: 10.1111/cts.13011
- Wang ZJ et al. (2021). Human biventricular electromechanical simulations on the progression of electrocardiographic and mechanical abnormalities in post-myocardial infarction. EP Europace 23(1):143-152. doi: 0.1093/europace/euaa405
- Corral-Acero J et al. (2020). The ‘Digital Twin’ to enable the vision of precision cardiology. European Heart Journal 41(48): 4556-4564. doi: 10.1093/eurheartj/ehaa159
- Li Z et al. (2020). General Principles for the Validation of Proarrhythmia Risk Prediction Models: An Extension of the CiPA In Silico Strategy. Clinical pharmacology and therapeutics 107(1):102-111. doi: 10.1002/cpt.1647
- Morissette P et al. (2020). Combining an in silico proarrhythmic risk assay with a tPKPD model to predict QTc interval prolongation in the anesthetized guinea pig assay. 390:114883. doi: 10.1016/j.taap.2020.114883
- Viceconti M et al. (2020). In silico trials: Verification, validation and uncertainty quantification of predictive models used in the regulatory evaluation of biomedical products. Methods (In Press) doi: 10.1016/j.ymeth.2020.01.011
- Delaunois A et al. (2019). An initial company experience gained from using preclinical CiPA testing paradigm in a drug discovery setting: Challenges still lying ahead. Journal of Pharmacological and Toxicological Methods 99:106595. doi: 10.1016/j.vascn.2019.05.098
- Ledezma CA et al. (2019). A modeling and machine learning approach to ECG feature engineering for the detection of ischemia using pseudo-ECG. PLoS One 14(8): e0220294. doi: 10.1371/journal.pone.0220294
- Lyon A et al. (2019). Improving the clinical understanding of hypertrophic cardiomyopathy by combining patient data, machine learning and computer simulations: A case study. Morphologie 103(343):169–179. doi: 10.1016/j.morpho.2019.09.001
- Mincholé A et al. (2019). Machine learning in the electrocardiogram. Journal of Electrocardiology 57:61-64. doi: 10.1016/j.jelectrocard.2019.08.008
- Mincholé A et al. (2019). MRI-Based Computational Torso/Biventricular Multiscale Models to Investigate the Impact of Anatomical Variability on the ECG QRS Complex. Frontiers in Physiology 10:1103. doi: 10.3389/fphys.2019.01103
- Martinez-Navarro H et al. (2019). High arrhythmic risk in antero-septal acute myocardial ischemia is explained by increased transmural reentry occurrence. Scientific Reports 9:16803. doi: 10.1038/s41598-019-53221-2
- Mincholé A and Rodriguez B (2019). Artificial intelligence for the electrocardiogram. Nature Medicine 25:22-23. doi: 10.1038/s41591-018-0306-1
- Morissette P et al. (2019). Impact of in vitro ion channel protocols when assessing cardiac pro-arrhythmic risk with in silico models. Journal of Pharmacological and Toxicological Methods 99:106595. doi: 10.1016/j.vascn.2019.05.103
- Passini E et al. (2019). Human in silico trials on drug-induced changes in electrophysiology and calcium dynamics using the virtual assay software. Journal of Pharmacological and Toxicological Methods 99:106595. doi: 10.1016/j.vascn.2019.05.104
- Passini E et al. (2019). Drug-induced shortening of the electromechanical window is an effective biomarker for in silico prediction of clinical risk of arrhythmias. British journal of pharmacology 176(19):3819-3833. doi: 10.1111/bph.14786
- Qu Y et al. (2019). Pro-arrhythmic risk assessment with a population model of human ventricular myocyte action potentials. Journal of Pharmacological and Toxicological Methods 99:106595. doi: 10.1016/j.vascn.2019.05.105
- Tomek J et al. (2019). Development, calibration, and validation of a novel human ventricular myocyte model in health, disease, and drug block. eLIFE 8:e48890. doi: 10.7554/eLife.48890
- Tomek J et al. (2019). β-Adrenergic Receptor Stimulation and Alternans in the Border Zone of a Healed Infarct: An ex vivo Study and Computational Investigation of Arrhythmogenesis. Frontiers in Physiology 10:350. doi: 10.3389/fphys.2019.00350
- Zhou X et al. (2019). Investigating the complex arrhythmic phenotype caused by the gain-of-function mutation KCNQ1-G229D. Frontiers in Physiology 10:259. doi: 10.3389/fphys.2019.00259
- Briant LJB et al. (2018). δ-cells and β-cells are electrically coupled and regulate α-cell activity via somatostatin. The Journal of physiology 596(2):197-215. doi: 10.1113/JP274581
- Cardone-Noott L et al. (2018). Strategies of data layout and cache writing for input-output optimization in high performance scientific computing: Applications to the forward electrocardiographic problem. PLOS ONE 13(8):e0202410. doi: 10.1371/journal.pone.0202410
- Grandi E et al. (2018). Editorial: Safety Pharmacology - Risk Assessment QT Interval Prolongation and Beyond. Frontiers in Physiology 9:678. doi: 10.3389/fphys.2018.00678
- Muszkiewicz A et al. (2018). From ionic to cellular variability in human atrial myocytes: an integrative computational and experimental study. American journal of physiology. Heart and circulatory physiology 314(5):H895-H916. doi: 10.1152/ajpheart.00477.2017
- Lawson BA et al. (2018). Unlocking data sets by calibrating populations of models to data density: A study in atrial electrophysiology. Science advances 4(1):e1701676. doi: 10.1126/sciadv.1701676
- Lawson BA et al. (2018). Slow Recovery of Excitability Increases Ventricular Fibrillation Risk as Identified by Emulation. Frontiers in Physiology 8:597. doi: 10.3389/fphys.2018.01114
- Lei CL et al. (2018). Beta-cell hubs maintain Ca2+ oscillations in human and mouse islet simulations. Islets 10(4):151–167. doi: 10.1080/19382014.2018.1493316
- Lyon A et al. (2018). Computational techniques for ECG analysis and interpretation in light of their contribution to medical advances. Journal of the Royal Society, Interface 15(138). doi: 10.1098/rsif.2017.0821
- Lyon A et al. (2018). Distinct ECG Phenotypes Identified in Hypertrophic Cardiomyopathy Using Machine Learning Associate With Arrhythmic Risk Markers. Frontiers in Physiology 9:213. doi: 10.3389/fphys.2018.00213
- Lyon A et al. (2018). Electrocardiogram phenotypes in hypertrophic cardiomyopathy caused by distinct mechanisms: apico-basal repolarization gradients vs. Purkinje-myocardial coupling abnormalities. EP Europace 20(3):102–112. doi: 10.1093/europace/euy226
- Zhou X et al. (2018). In silico evaluation of arrhythmia. Current Opinion in Physiology 1:95-103. doi: 10.1016/j.cophys.2017.11.003
- Briant LJB et al. (2017). Functional identification of islet cell types by electrophysiological fingerprinting. Journal of the Royal Society, Interface 14(128). doi: 10.1098/rsif.2016.0999
- Britton OJ et al. (2017). The Electrogenic Na+/K+ Pump Is a Key Determinant of Repolarization Abnormality Susceptibility in Human Ventricular Cardiomyocytes: A Population-Based Simulation Study. Frontiers in Physiology 8:278. doi: 10.3389/fphys.2017.00278
- Britton OJ et al. (2017). Quantitative Comparison of Effects of Dofetilide, Sotalol, Quinidine, and Verapamil between Human Ex vivo Trabeculae and In silico Ventricular Models Incorporating Inter-Individual Action Potential Variability. Frontiers in Physiology 8:597. doi: 10.3389/fphys.2017.00597
- Bueno-Orovio A and Burrage K. (2017). Exact solutions to the fractional time-space Bloch-Torrey equation for magnetic resonance imaging. 52:91-109. doi: 10.1016/j.cnsns.2017.04.013
- Paci M et al. (2017). Phenotypic variability in LQT3 human induced pluripotent stem cell-derived cardiomyocytes and their response to antiarrhythmic pharmacologic therapy: An in silico approach. Heart Rhythm 14:1704. doi: 10.1016/j.hrthm.2017.07.026
- Passini E et al. (2017). Human in silico drug trials demonstrate higher accuracy than animal models in predicting clinical pro-arrhythmic cardiotoxicity. Frontiers in Physiology 8:668. doi: 10.3389/fphys.2017.00668
- Sánchez C et al. (2017). Atrial Fibrillation Dynamics and Ionic Block Effects in Six Heterogeneous Human 3D Virtual Atria with Distinct Repolarization Dynamics. Frontiers in bioengineering and biotechnology 5:29. doi: 10.3389/fbioe.2017.00029
- Tomek J et al. (2017). β-Adrenergic receptor stimulation inhibits proarrhythmic alternans in postinfarction border zone cardiomyocytes: a computational analysis. American journal of physiology. Heart and circulatory physiology 313(2):H338-H353. doi: 10.1152/ajpheart.00094.2017
- Tixier E et al. (2017). Modelling variability in cardiac electrophysiology: a moment-matching approach. Journal of the Royal Society, Interface 14(133). doi: 10.1098/rsif.2017.0238
- Zacur E et al. (2017). MRI-Based Heart and Torso Personalization for Computer Modeling and Simulation of Cardiac Electrophysiology. In: Cardoso M. et al. (eds) Imaging for Patient-Customized Simulations and Systems for Point-of-Care Ultrasound. BIVPCS 2017, POCUS 2017. Lecture Notes in Computer Science, vol 10549. Springer, Cham doi: 10.1007/978-3-319-67552-7_8