Exercises to enhance critical analysis skills and improve your ability to evaluate scientific literature.
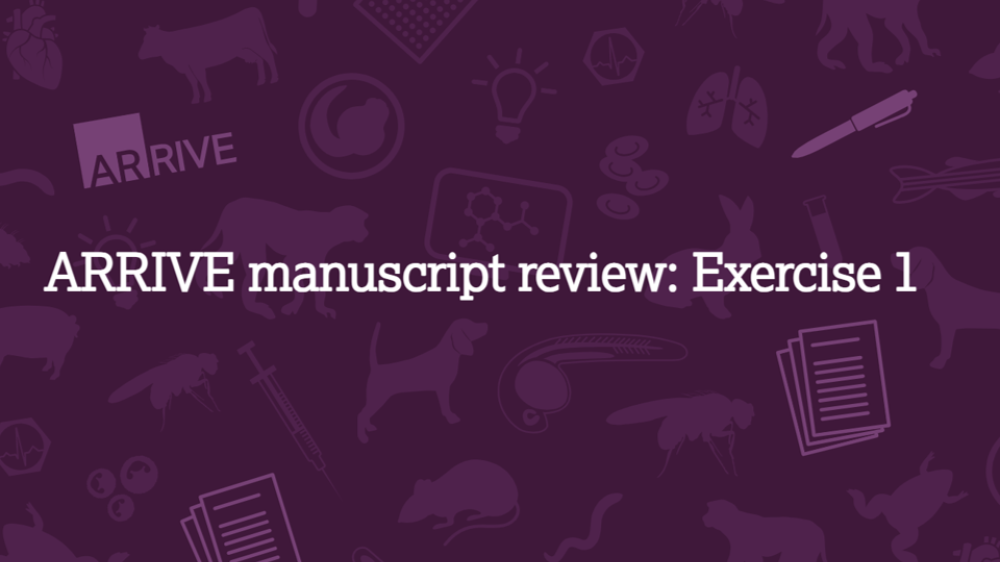
Exercises to enhance critical analysis skills and improve your ability to evaluate scientific literature.
Reasons for conducting a pilot study and what you can do with the results.
Practical guidance and examples of strategies that can be used to mask group allocation in animal studies.
A webinar explaining the importance of using both sexes in animal experiments and things to consider when planning studies.
Learn how our online EDA tool can help you plan rigorous experiments.
An introduction to systematic reviews, a powerful method used to assess accumulating evidence related to a specific research question.
Reporting guidelines for animal experiments, and additional resources to support their use.
An online tool to guide researchers through designing experiments involving the use of animals.
Tips to avoid common pitfalls when describing your experimental design and methodology in a funding proposal.
An online resource to help you perform systematic reviews and meta-analyses.
A webinar introducing the revised ARRIVE guidelines, the items in the ARRIVE Essential 10, and relevant resources.
An introduction for early career and postdoctoral researchers, applicable to both in vivo and in vitro experiments.
A webinar for in vivo researchers or students with limited or no experience of systematic reviews.
Dr Simon Bate, from Statistical Sciences, GSK, covers a few questions about statistics and the 3Rs that he regularly encounters.
A recorded talk highlighting where bias can be introduced into experiments and the implications for results.
A recorded talk explaining the dangers of underpowered experiments and how good design can increase the chances of finding reliable results.
Discussing key considerations when determining the effect size for a study, including why this is important for sample size calculations.
A video overview of common practices in preclinical research, and specific actions that can be taken to improve its robustness.
Videos from our May 2018 workshop on experimental design for panel members of the NC3Rs, BBSRC, CRUK, MRC and Wellcome Trust.
Things to consider when designing your animal experiments.